Main message
Framework for drawing causal inference from observational studies:
- Treatment groups
- Patient-level outcome
- Summary measure of outcome
- Difference in outcome among groups
- Attribution to group membership
- Factors to control
- Factors we should not control
We published a study on timing alternatives for hip fracture surgery in CMAJ https://doi.org/10.1503/cmaj.171512
We offer two new estimates: projected mortality and attributable deaths.
How did we argue our case that early surgery improves survival after hip fracture?
- We state we are concerned with a policy question, not an associational question. We ask what mortality should be expected if timing policy for hip fracture surgery would change. An associational question is whether mortality varies in patients with various surgical times.
- We acknowledge that answering the policy question with observational data involves counterfactuals, such as expected mortality should all patients had early surgery.
- We stratify observations on factors that block biasing influences from known factors. We use the back-door criterion to find stratification factors in a evidence-informed causal diagram.
- We observe stratification factors have constant values within each stratum. We reason then difference in mortality by timing is unbiased in each strata, because conditioning on statification factors prevents other factors from producing covariation in mortality and timing.
- We average stratum-specific risk differences between timing alternatives to find difference in mortality attributable to a policy change.
- We conclude that if all hip fracture surgeries in Canada were within 2 days, then 8 more patients would survive to hospital discharge, for every 1000 surgeries.
CMAJ August 07, 2018 190 (31) E923-E932

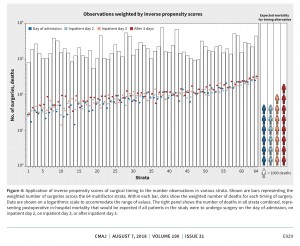